About
이번 포스트에서는 Tensorflow를 이용하여 Deep Neural Networks를 구현하는 법을 간단히 알아보도록 하고, 어떻게 하면 코드 복사 붙여넣기 없이 할 수 있을까에 대해서 생각해보고 구현한 것을 공유하고자 한다.
특히 찾아보면 간단한 예제를 통해서 개념들을 설명하는 경우는 많지만 Practical한 예제를 사용한 경우는 드물어서 필자는 조금 더 Practical하게 작성하고자 노력해봤다.
다만 물론 필자도 경험이 많은 것이 아니어서, 아래의 예들이 좋은 코드 패턴은 아닐 수 있음에 양해를 구하며, 만약 더 좋은 생각이 나 궁금한 점은 댓글을 통해서 꼭 알려주시길 부탁드린다.
만약 Neural Network에 대해서 잘 모르신다면 아래 링크들을 확인하시길 1.
인공신경망에 대한 이해(Part 1 - Feedforward Propagation) 2.인공신경망에 대한 이해(Part 2 - Back Propagation)
Tensorflow를 이용한 DNN 실습
연습으로 MNIST Digit 이미지를 이용하도록 하자.
코드에서는 주석을 보며 생각흐름을 따라오면 된다.
import numpy as np
import tensorflow as tf
import matplotlib.pyplot as plt
import random
from keras.utils import np_utils
mnist = tf.keras.datasets.mnist
# mnist dataset을 load한다.
(x_train, y_train),(x_test, y_test) = mnist.load_data()
# float로 변환하고 minmax 스케일링을 한다. 이는 이미지 전처리의 가장 보편적인 방법 중 하나이다.
x_train = x_train.reshape(60000, 784).astype('float32') / 255.0
x_test = x_test.reshape(10000, 784).astype('float32') / 255.0
print(x_train.shape, x_train.dtype)
# y 값을 one-hot-encoding로 변환해준다.
y_unique_num = len(np.unique(y_train))
y_train = np_utils.to_categorical(y_train, y_unique_num)
y_test = np_utils.to_categorical(y_test, y_unique_num)
y_train[:5]
# test로 이미지를 한번 출력해보자.
r = random.randint(0, x_train.shape[0] - 1)
plt.imshow(
x_train[r].reshape(28, 28),
cmap="Greys",
interpolation="nearest" # 중간에 비어있는 값 처리
)
plt.show()
먼저 클래스를 사용하지 않고 구현해보자.
아래는 Graph를 만드는 코드다.
혹시 placeholder, Variable 등 기본적인 함수에 대해서 잘 모른다면,
# input data를 위한 공간(placeholder)를 만든다.
X = tf.placeholder(tf.float32, shape=[None, 28*28*1])
# label data를 위한 공간도 만든다.
y = tf.placeholder(tf.float32, shape=[None, 10])
# layer 1
W1 = tf.Variable(tf.random_normal([28*28*1, 10]))
b1 = tf.Variable(tf.random_normal([10]))
# 이번 예제에서는 activation 함수로는 sigmoid를 사용하기로 하자.
layer1 = tf.sigmoid(tf.matmul(X, W1) + b1)
# layer 2
W2 = tf.Variable(tf.random_normal([10, 20]))
b2 = tf.Variable(tf.random_normal([20]))
layer2 = tf.sigmoid(tf.matmul(layer1, W2) + b2)
# layer 3
W3 = tf.Variable(tf.random_normal([20, 20]))
b3 = tf.Variable(tf.random_normal([20]))
layer3 = tf.sigmoid(tf.matmul(layer2, W3) + b3)
# layer 4
W4 = tf.Variable(tf.random_normal([20, 10]))
b4 = tf.Variable(tf.random_normal([10]))
hypothesis = tf.nn.softmax(tf.matmul(layer3, W4) + b4)
cost = tf.reduce_mean(-tf.reduce_sum(y * tf.log(hypothesis), axis=1))
train = tf.train.GradientDescentOptimizer(learning_rate=0.01).minimize(cost)
prediction = tf.argmax(hypothesis, 1)
is_correct = tf.equal(prediction, tf.argmax(y, 1))
accuracy = tf.reduce_mean(tf.cast(is_correct, tf.float32))
그리고 세션을 이용해서 학습해보자.
sess = tf.Session()
sess.run(tf.global_variables_initializer())
batch_size = 200
epoch = 100
for step in range(epoch):
total_batch = int(len(x_train)/batch_size)
c_avg = 0
for i in range(total_batch):
batch_x = x_train[batch_size*i : batch_size*(i+1)]
batch_y = y_train[batch_size*i : batch_size*(i+1)]
c, _ = sess.run([cost, train], feed_dict={X: batch_x, y: batch_y})
c_avg = c_avg + (c/total_batch)
if step % 10 == 0:
print(step, c_avg)
print(sess.run(accuracy, feed_dict={X: x_test, y: y_test}))
필자가 이 네트워크로 얻은 Accuracy값은 0.7291이었다. 그렇다면 이제 네트워크를 바꿔가며 하이퍼패러미터 튜닝을 시도해야할텐데, 그때마다 위의 Graph코드를 복사해서 붙여넣고 중간에 layer들은 변경한다거나 해야한다.
코드도 지저분해지고 자유도가 엄청 떨어지는 이 문제점을 해결하기 위해서 아래처럼 모델은 Class로 Train은 함수로 따로 구현해봤다.
코드가 많이 복잡해보이는데, 그 이유는 크게 4가지이다.
- Model은 Graph를 만드는 역할만 수행하고 Session과 결합하지 않았다.
- Model을 빌드할 때 자유롭게 미리 config에서 설정한 layer, neuron의 개수, initializer, activation 등을 적용할 수 있게 하였다.
- 각 Layer마다 사용된 variable을 가져올 수 있게 하였다.
- Tensorboard에도 기록될 수 있게 하였다.
이번 예제에서는 구현하지는 않았지만, activation이나 initializer 등을 넘어서 dropout 등도 응용해서 적용하면 된다.
그렇게되면 장점은
- 내부 layer 등을 달리한 모델 m1, m2를 객체화하고 학습은 같은 train() 함수를 이용해서 진행할 수 있어서 객체 내부에 중복된 train 함수를 들고있을 필요가 없다.
- 더 큰 네트워크를 만들기 용이하다.
이제 코드로 살펴보자.
먼저 사용법을 살펴보고 나머지들을 설명하도록 하겠다.
# input 데이터가 가진 feature 개수
n_features = x_train.shape[1]
# label 개수
n_class = len(y_train[0])
# model build를 위한 config를 만든다.
config = {
"name" : "dnn_model", # 나중에 tensorboard를 확인하면 여기서 정한 이름으로 graph가 만들어진다.
"n_features" : n_features,
"n_class" : n_class,
"n_li" : [n_features, 1000, 1000, 1000, n_class], # input부터 output사이의 hidden layer neuron 개수들을 리스트형식으로 적어준다.
"initializer_li" : ["random_normal", "random_normal", "random_normal", "random_normal"], # 각 레이어마다 Variable들이 사용할 initializer를 적어준다. 코드에서는 random_normal, xavier 두 가지 경우만을 고려하였다.
"activation_li" : ["sigmoid", "sigmoid", "sigmoid", None]
# 각 레이어별로 뉴론에서 사용할 activation 함수를 적어준다. 코드에서는 sigmoid와 relu 두 가지 경우만을 고려하였다.
}
# 객체를 만들자
dnn_model = DNNModel(config)
# train함수에 만든 graph와 x_train, y_train을 넣어준다. epoch, lr, batch_size 등도 여기서 변경하며 실험해볼 수 있다.
train(dnn_model, x_train, y_train, epoch=15)
# accuracy, predict도 모델과 샘플 데이터들을 넣어주면 된다. 참고로 위에서 선언한 네트워크로 필자는 accuracy가 0.8로 나왔다.
accuracy(dnn_model, x_test, y_test), predict(dnn_model, x_test)
Model Class
class DNNModel:
def __init__(self, config):
self.config = config # 위에서 넣어준 config를 객체 내부에 저장하자.
self.endpoints = {} # layer마다 사용한 variable을 저장할 공간을 만든다.
self.graph = tf.Graph() # graph 정보를 train에서 session을 연결할 때 사용해야하므로 역시 객체에 저장해준다.
def build_net(self, x_placeholder, y_placeholder):
with self.graph.as_default(): # 위에서 선언한 graph안에 빌드를 한다.
with tf.variable_scope(self.config["name"]): # tensorboard에서 확인하기 좋고, debugging에 유리하도록 name을 설정해준다.
self.X = x_placeholder # 모델 클래스 자체가 blackbox처럼 만들기위해서 x_placeholder는 외부에서 주입받도록 하였다. input to output 매핑이 가능하도록..
self.y = y_placeholder # 마찬가지로 위부(train 함수)에서 주입을 반든다.
layer_output_li = []
# 항상 다음 layer에서 activation 함수를 통과할 때는 직전 layer에서 activation을 통과해서 나온 값과 현재 layer의 weight 및 bias와 연산을 진행하게된다.
# 그러므로 각 layer output은 리스트로 저장해서 필요시 사용하도록 한다.
for idx, n in enumerate(self.config["n_li"][:-1]):
with tf.name_scope("Layer_" + str(idx)) as scope:
previous_dim = self.config["n_li"][idx]
next_dim = self.config["n_li"][idx + 1]
# 아래의 shape은 중간의 weights matrix와 bias의 shape를 위해 필요하다.
shape = [previous_dim, next_dim]
# 이전 layer output을 가져오도록 하자.
pre_layer_output = layer_output_li[-1] if idx > 0 else self.X
self.__set_weight_and_bias(idx, shape)
layer = self.__set_layer_endpoint(idx, pre_layer_output)
layer_output_li.append(layer)
with tf.name_scope("Cost") as scope:
self.cost = tf.reduce_mean(tf.nn.softmax_cross_entropy_with_logits_v2(logits=self.logits, labels=self.y))
cost_sum = tf.summary.scalar("Cost", self.cost)
self.predict = tf.argmax(self.logits, 1)
correct_prediction = tf.equal(tf.argmax(self.logits, 1), tf.argmax(self.y, 1))
self.accuracy = tf.reduce_mean(tf.cast(correct_prediction, tf.float32))
def __set_weight_and_bias(self, idx, shape):
# random_normal과 xavier만 만들었지만, 필요하면 if문을 추가하면된다.
if self.config["initializer_li"][idx] == "random_normal":
self.endpoints["W_" + str(idx)] = tf.Variable(tf.random_normal(shape), name = "W_" + str(idx))
elif self.config["initializer_li"][idx] == "xavier":
self.endpoints["W_" + str(idx)] = tf.get_variable("W_" + str(idx), shape=shape,
initializer=tf.contrib.layers.xavier_initializer())
self.endpoints["b_" + str(idx)] = tf.Variable(tf.random_normal(shape[1:]), name = "b_" + str(idx))
W_hist = tf.summary.histogram("W_hist_" + str(idx), self.endpoints["W_" + str(idx)])
b_hist = tf.summary.histogram("b_hist_" + str(idx), self.endpoints["b_" + str(idx)])
def __set_layer_endpoint(self, idx, pre_layer_output):
W = self.endpoints["W_" + str(idx)]
b = self.endpoints["b_" + str(idx)]
if idx + 1 == len(self.config["n_li"][:-1]):
self.logits = tf.matmul(pre_layer_output, W) + b
layer_hist = tf.summary.histogram("Layer_hist_" + str(idx), self.logits)
return self.logits
# weight & bias와 마찬가지로 필요하면 sigmoid, relu 이외에도 추가하면 된다.
if self.config["activation_li"][idx] == "sigmoid":
self.endpoints["layer_" + str(idx)] = tf.sigmoid(tf.matmul(pre_layer_output, W) + b)
elif self.config["activation_li"][idx] == "relu":
self.endpoints["layer_" + str(idx)] = tf.nn.relu(tf.matmul(pre_layer_output, W) + b)
layer_hist = tf.summary.histogram("Layer_hist_" + str(idx), self.endpoints["layer_" + str(idx)])
return self.endpoints["layer_" + str(idx)]
Train function
def train(model, X_train, y_train, lr=1e-4, epoch=15, batch_size=200):
# 모델의 그래프 안에 build하지 않으면 찾을 수 없다고 오류가 발생한다.
with model.graph.as_default():
x_placeholder = tf.placeholder(tf.float32, shape=[None, model.config["n_features"]], name="X")
y_placeholder = tf.placeholder(tf.float32, shape=[None, model.config["n_class"]], name="y")
# graph에 build
model.build_net(x_placeholder, y_placeholder)
# Session이 정확히 특정한 graph에 연결을 하기 때문에 각 객체간에 엇갈릴 일이 없다.
with tf.Session(graph=model.graph) as sess:
train_op = tf.train.AdamOptimizer(learning_rate=lr).minimize(model.cost)
init = tf.global_variables_initializer()
merged_summary = tf.summary.merge_all()
writer = tf.summary.FileWriter("./logs", sess.graph)
sess.run(init)
for step in range(epoch):
total_batch = int(len(X_train)/batch_size)
c_avg = 0
for i in range(total_batch):
batch_x = X_train[batch_size*i : batch_size*(i+1)]
batch_y = y_train[batch_size*i : batch_size*(i+1)]
summary, c, _ = sess.run([merged_summary, model.cost, train_op],
feed_dict={model.X: batch_x, model.y: batch_y})
c_avg = c_avg + (c/total_batch)
writer.add_summary(summary, i)
print(step, c_avg)
saver = tf.train.Saver()
# predict나 accuracy도 model 밖에서 접근하므로 use uninitialized weights 오류를 피하려면 checkpoint를 저장하고 불러쓰는 방법을 써야했다.
saver.save(sess, './checkpoint/' + model.config["name"] + '.chkp')
Predict and accuracy function
def predict(model, x_test):
with tf.Session(graph=model.graph) as sess:
saver = tf.train.Saver()
saver.restore(sess, './checkpoint/' + model.config["name"] + '.chkp')
return sess.run([model.predict], feed_dict={model.X : x_test})
def accuracy(model, x_test, y_test):
with tf.Session(graph=model.graph) as sess:
saver = tf.train.Saver()
saver.restore(sess, './checkpoint/' + model.config["name"] + '.chkp')
return sess.run([model.accuracy], feed_dict={model.X : x_test, model.y : y_test})
마지막으로 학습한 Endpoints를 확인하고 싶다면?
dnn_model.endpoints
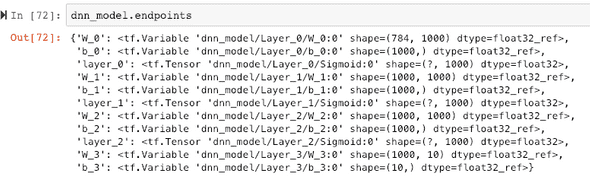
참고로 아래처럼 config를 하면 accuracy는 0.9749까지 올라간다.(이 network가 최고라는 것은 결코 아니니 오해하지 마시길)
n_features = x_train.shape[1]
n_class = len(y_train[0])
config = {
"name" : "dnn_model",
"n_features" : n_features,
"n_class" : n_class,
"n_li" : [n_features, 1000, 1000, 1000, n_class],
"initializer_li" : ["xavier", "xavier", "xavier", "xavier"],
"activation_li" : ["relu", "relu", "relu", None]
}
dnn_model = DNNModel(config)
train(dnn_model, x_train, y_train, epoch=15)
전체 코드는 github에도 올려놨으니 필요하신분은 확인하시길..
위에서도 설명했지만 이 방법이 좋은 방법인지 필자도 알 수는 없다. 적어도 필자의 목적은 이룬 코드 패턴이어서 소개를 하였는데, 부디 도움이 되길 바란다.